Are you drowning in a sea of acronyms—KPI, OKR, ROI, CPC, ROAS, MRR? It's a cacophony of letters, a metrics meltdown. How do you make sense of it all? Let me help you navigate two categories of metrics - KPIs and OKRs.
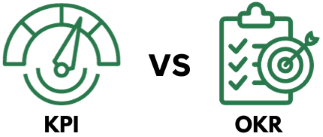
Let's start at the beginning, shall we? Key Performance Indicators (KPIs) are the metrics that monitor the performance of specific aspects of your business. Imagine them as the dashboard of your car, telling you how fast you're going or how much fuel you have left. KPIs measure the tangible outcomes like sales, customer satisfaction, and productivity. Want to know how many products you sold last month? Check the KPIs. Curious about customer satisfaction? The KPIs will have that information too.
But KPIs are not a one-size-fits-all solution. Sales, marketing, customer support — each department has its own set of vital signs. It's about tailoring the KPIs to your business DNA, ensuring they're the true pulse of your operations.
OBJECTIVES AND KEY RESULTS (OKRs)
Now, enter Objectives and Key Results (OKRs), the cool, trendy cousin. OKRs are ambitious, goal-oriented frameworks designed to drive organizational alignment and success. OKRs are the dreamers, the visionaries. They set the stage for the big picture, outlining ambitious objectives that push the boundaries of what's possible. The key results, like the milestones on a road trip, help you track progress toward those lofty goals.
An Objective (O) might be to grow from $75 million to $100 million dollars in revenue. A Key Result (KR) for that particular revenue objective might be quantitative: improve customer retention by 10% while another KR might be qualitative such as: create a roadmap of Sales and Marketing strategies to increase revenue.